Invited Talks
The A.I. industry has created new jobs that have been essential to the real-world deployment of intelligent systems. These new jobs typically focus on labeling data for machine learning models or having workers complete tasks that A.I. alone cannot do. Human labor with A.I. has powered a futuristic reality where self-driving cars and voice assistants are now commonplace. However, the workers powering our A.I. industry are often invisible to consumers. Together, this has facilitated a reality where these invisible workers are often paid below minimum wage and have limited career growth opportunities. In this talk, I will present how we can design a future of work for empowering the invisible workers behind our A.I. I propose a framework that transforms invisible A.I. labor into opportunities for skill growth, hourly wage increase, and facilitates transitioning to new creative jobs that are unlikely to be automated in the future. Taking inspiration from social theories on solidarity and collective action, my framework introduces two new techniques for creating career ladders within invisible A.I. labor: a) Solidarity Blockers, computational methods that use solidarity to collectively organize workers to help each other to build new skills while completing invisible labor; and b) Entrepreneur Blocks, computational techniques that, inspired from collective action theory, guide invisible workers to create new creative solutions and startups in their communities. I will present case-studies showcasing how this framework can drive positive social change for the invisible workers in our A.I. industry. I will also connect how governments and civic organizations in Latin America and U.S. rural states can use the proposed framework to provide new and fair job opportunities. In contrast to prior research that focused primarily on improving A.I., this talk will empower you to create a future that has solidarity with the invisible workers in our A.I. industry.
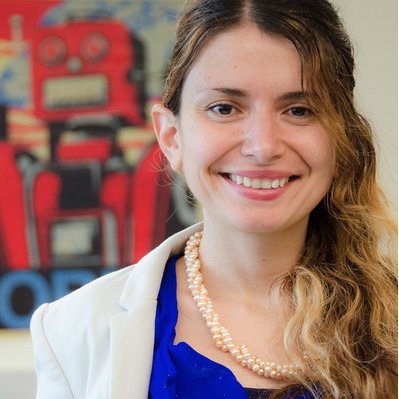
Saiph Savage
Saiph Savage is the co-director of the Civic Innovation Lab at the National Autonomous University of Mexico (UNAM) and director of the HCI Lab at West Virginia University. Her research involves the areas of Crowdsourcing, Social Computing and Civic Technology. For her research, Saiph has been recognized as one of the 35 Innovators under 35 by the MIT Technology Review. Her work has been covered in the BBC, Deutsche Welle, and the New York Times. Saiph frequently publishes in top tier conferences, such as ACM CHI, AAAI ICWSM, the Web Conference, and ACM CSCW, where she has also won honorable mention awards. Saiph has received grants from the National Science Foundation, as well as funding from industry actors such as Google, Amazon, and Facebook Research. Saiph has opened the area of Human Computer Interaction in West Virginia University, and has advised Governments in Latin America to adopt Human Centered Design and Machine Learning to deliver smarter and more effective services to citizens. Saiph’s students have obtained fellowships and internships in both industry (e.g., Facebook Research, Twitch Research, and Microsoft Research) and academia (e.g., Oxford Internet Institute.) Saiph holds a bachelor's degree in Computer Engineering from UNAM, and a Ph.D. in Computer Science from the University of California, Santa Barbara. Dr.Savage has also been a Visiting Professor in the Human Computer Interaction Institute at Carnegie Mellon University (CMU).
Successful technological fields have a moment when they become pervasive, important, and noticed. They are deployed into the world and, inevitably, something goes wrong. A badly designed interface leads to an aircraft disaster. A buggy controller delivers a lethal dose of radiation to a cancer patient. The field must then choose to mature and take responsibility for avoiding the harms associated with what it is producing. Machine learning has reached this moment.
In this talk, I will argue that the community needs to adopt systematic approaches for creating robust artifacts that contribute to larger systems that impact the real human world. I will share perspectives from multiple researchers in machine learning, theory, computer perception, and education; discuss with them approaches that might help us to develop more robust machine-learning systems; and explore scientifically interesting problems that result from moving beyond narrow machine-learning algorithms to complete machine-learning systems.
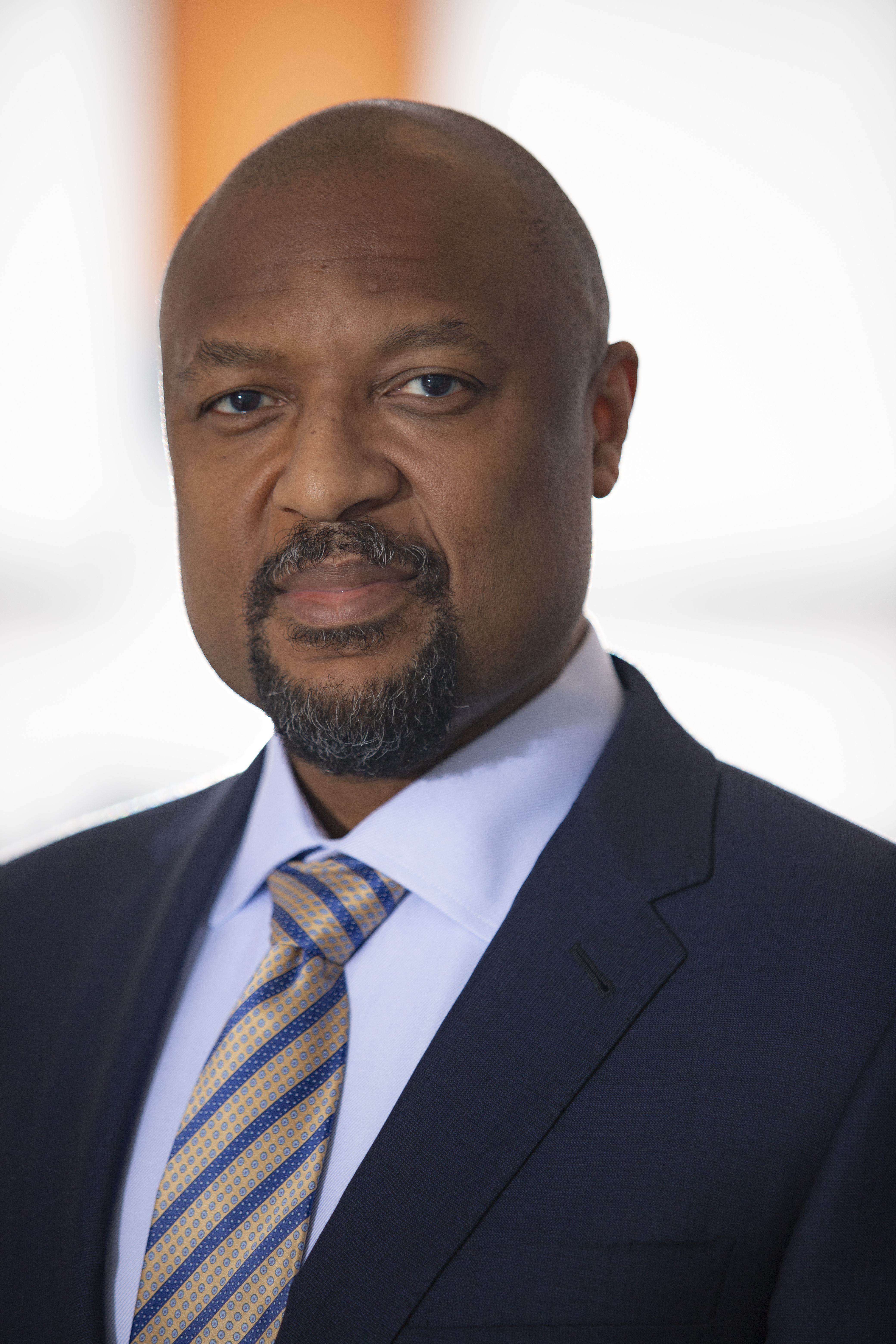
Charles Isbell
Dr. Charles Isbell received his bachelor's in Information and Computer Science from Georgia Tech, and his MS and PhD at MIT's AI Lab. Upon graduation, he worked at AT&T Labs/Research until 2002, when he returned to Georgia Tech to join the faculty as an Assistant Professor. He has served many roles since returning and is now The John P. Imlay Jr. Dean of the College of Computing.
Charles’s research interests are varied but the unifying theme of his work has been using machine learning to build autonomous agents who engage directly with humans. His work has been featured in the popular press, congressional testimony, and in several technical collections.
In parallel, Charles has also pursued reform in computing education. He was a chief architect of Threads, Georgia Tech’s structuring principle for computing curricula. Charles was also an architect for Georgia Tech’s First-of-its’s-kind MOOC-supported MS in Computer Science. Both efforts have received international attention, and been presented in the academic and popular press.
In all his roles, he has continued to focus on issues of broadening participation in computing, and is the founding Executive Director for the Constellations Center for Equity in Computing. He is an AAAI Fellow and a Fellow of the ACM. Appropriately, his citation for ACM Fellow reads “for contributions to interactive machine learning; and for contributions to increasing access and diversity in computing”.
Many animals are born with impressive innate capabilities. At birth, a spider can build a web, a colt can stand, and a whale can swim. From an evolutionary perspective, it is easy to see how innate abilities could be selected for: Those individuals that can survive beyond their most vulnerable early hours, days or weeks are more likely to survive until reproductive age, and attain reproductive age sooner. I argue that most animal behavior is not the result of clever learning algorithms, but is encoded in the genome. Specifically, animals are born with highly structured brain connectivity, which enables them to learn very rapidly. Because the wiring diagram is far too complex to be specified explicitly in the genome, it must be compressed through a “genomic bottleneck,” which serves as a regularizer. The genomic bottleneck suggests a path toward architectures capable of rapid learning.
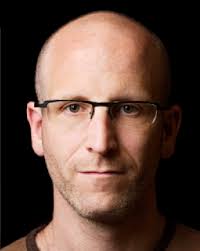
Anthony M Zador
Anthony Zador is the Alle Davis Harrison Professor of Biology and former Chair of Neuroscience at Cold Spring Harbor Laboratory (CSHL). His laboratory focuses on three interrelated areas. First they study neural circuits underlying decisions about auditory and visual stimuli, using rodents as a model system. Second, they have pioneered a new class of technologies for determining the wiring diagram of a neural circuit. This approach--MAPseq and BARseq---converts neuronal wiring into a form that can be read out by high-throughput DNA sequencing. Finally, they are applying insights from neuroscience to artificial intelligence, attempting to close the gap between the capabilities of natural intelligence and the more limited capacities of current artificial systems.
Zador is a founder of the Cosyne conference, which brings together theoretical and experimental neuroscientists; and of the NAISys conference, which brings together neuroscientists and researchers in artificial intelligence. He has also launched a NeuroAI Scholars initiative at CSHL, a two-year program which helps early-stage researchers with a solid foundation in modern AI become fluent in modern neuroscience.
We will present cryptography inspired models and results to address three challenges that emerge when worst-case adversaries enter the machine learning landscape. These challenges include verification of machine learning models given limited access to good data, training at scale on private training data, and robustness against adversarial examples controlled by worst case adversaries.
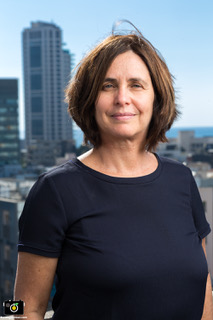
Shafi Goldwasser
Shafi Goldwasser is Director of the Simons Institute for the Theory of Computing, and Professor of Electrical Engineering and Computer Science at the University of California Berkeley. Goldwasser is also Professor of Electrical Engineering and Computer Science at MIT and Professor of Computer Science and Applied Mathematics at the Weizmann Institute of Science, Israel. Goldwasser holds a B.S. Applied Mathematics from Carnegie Mellon University (1979), and M.S. and Ph.D. in Computer Science from the University of California Berkeley (1984).
Goldwasser's pioneering contributions include the introduction of probabilistic encryption, interactive zero knowledge protocols, elliptic curve primality testings, hardness of approximation proofs for combinatorial problems, and combinatorial property testing.
Goldwasser was the recipient of the ACM Turing Award in 2012, the Gödel Prize in 1993 and in 2001, the ACM Grace Murray Hopper Award in 1996, the RSA Award in Mathematics in 1998, the ACM Athena Award for Women in Computer Science in 2008, the Benjamin Franklin Medal in 2010, the IEEE Emanuel R. Piore Award in 2011, the Simons Foundation Investigator Award in 2012, and the BBVA Foundation Frontiers of Knowledge Award in 2018. Goldwasser is a member of the NAS, NAE, AAAS, the Russian Academy of Science, the Israeli Academy of Science, and the London Royal Mathematical Society. Goldwasser holds honorary degrees from Ben Gurion University, Bar Ilan University, Carnegie Mellon University, Haifa University, University of Oxford, and the University of Waterloo, and has received the UC Berkeley Distinguished Alumnus Award and the Barnard College Medal of Distinction.
The impact of feedback control is extensive. It is deployed in a wide array of engineering domains, including aerospace, robotics, automotive, communications, manufacturing, and energy applications, with super-human performance having been achieved for decades. Many settings in learning involve feedback interconnections, e.g., reinforcement learning has an agent in feedback with its environment, and multi-agent learning has agents in feedback with each other. By explicitly recognizing the presence of a feedback interconnection, one can exploit feedback control perspectives for the analysis and synthesis of such systems, as well as investigate trade-offs in fundamental limitations of achievable performance inherent in all feedback control systems. This talk highlights selected feedback control concepts—in particular robustness, passivity, tracking, and stabilization—as they relate to specific questions in evolutionary game theory, no-regret learning, and multi-agent learning.
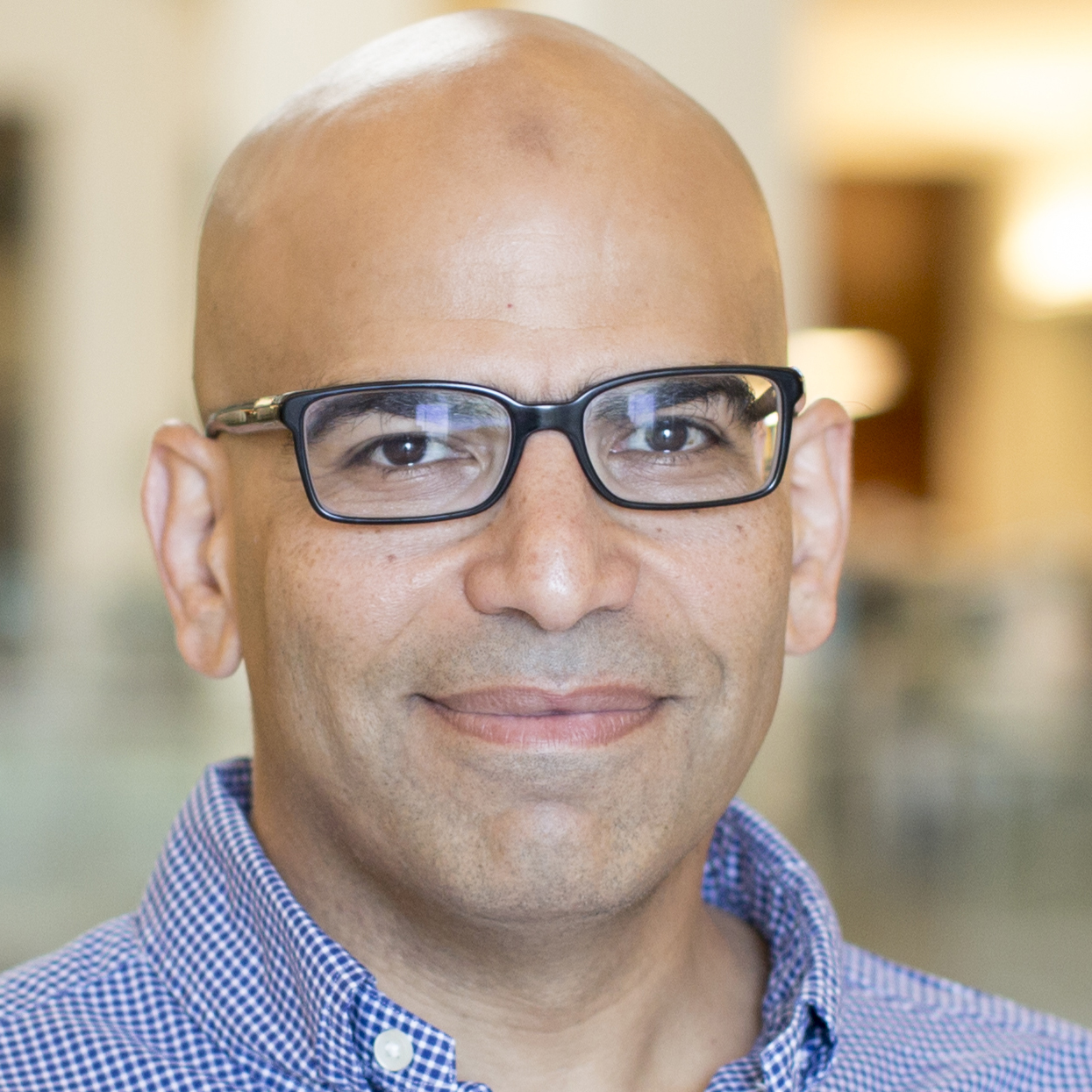
Jeff Shamma
Jeff S. Shamma is currently a Professor of Electrical and Computer Engineering at the King Abdullah University of Science and Technology (KAUST). At the end of the year, he will join the University of Illinois at Urbana-Champaign as the Department Head of Industrial and Enterprise Systems Engineering (ISE) and Jerry S. Dobrovolny Chair in ISE. Jeff received a Ph.D. in systems science and engineering from MIT in 1988. He is a Fellow of IEEE and IFAC, a recipient of the IFAC High Impact Paper Award, and a past semi-plenary speaker at the World Congress of the Game Theory Society. Jeff is currently serving as the Editor-in-Chief for the IEEE Transactions on Control of Network Systems.