Invited Talks
Duolingo is the most popular way to learn languages in the world. With over half a billion exercises completed every day, we have the largest dataset of people learning languages ever amassed. In this talk I will describe all the different ways in which we use AI to improve how well we teach and how to keep our learners engaged.
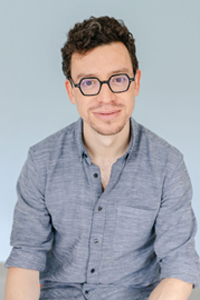
Luis von Ahn
Luis von Ahn is an entrepreneur and former computer science professor at Carnegie Mellon University who is considered one of the pioneers of crowdsourcing. He is known for co-inventing CAPTCHAs, being a MacArthur Fellow, and selling two companies to Google in his 20s.
He is currently the co-founder and CEO of Duolingo (NASDAQ: DUOL), a language-learning platform created to bring free language education to the world. With over 500 million users, it is now the most popular way to learn languages and the most downloaded education app in the world.
Luis has been named one of the 10 Most Brilliant Scientists by Popular Science Magazine, one of the 50 Best Brains in Science by Discover, one of the Top Young Innovators Under 35 by MIT Technology Review, one of the 100 Most Innovative People in Business by Fast Company Magazine, and in 2018 won the Lemelson-MIT Prize.
If data is power, this keynote asks what methodologies and frameworks, beyond measuring bias and fairness in ML, might best serve communities that are, otherwise, written off as inevitable ‘data gaps?’ To address this question, the talk applies design justice principles articulated in 2020 by scholar Costanza-Chock to the case of community-based organizations (CBOs) serving marginalized Black and Latinx communities in North Carolina. These CBOs, part of an 8-month study of community healthcare work, have become pivotal conduits for COVID-19 health information and equitable vaccine access. As such, they create and collect the so-called ‘sparse data’ of marginalized groups often missing from healthcare analyses. How might health equity—a cornerstone of social justice—be better served by equipping CBOs to collect community-level data and set the agendas for what to share and learn from the people that they serve? The talk will open with an analysis of the limits of ML models that prioritize the efficiencies of scale over attention to just and inclusive sampling. It will then examine how undertheorized investments in measuring bias and fairness in data and decision-making systems distract us from considering the value of collecting data with rather than for communities. Outlining an early learning theory proposed by Russian psychologist Lev Vygotsky (1978), the presentation will argue that focusing on the demands of collecting community members’ data and observing the social interactions that are computationally hard to measure but qualitatively invaluable to see are necessary to advance socially-just ML. The talk will conclude with recommendations for how to reorient computer science and machine learning to a more explicit theory and practice of data power-sharing.
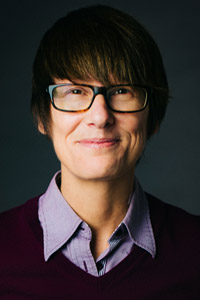
Mary L. Gray
Mary L. Gray is Senior Principal Researcher at Microsoft Research and Faculty Associate at Harvard University’s Berkman Klein Center for Internet and Society. She maintains a faculty position in the Luddy School of Informatics, Computing, and Engineering with affiliations in Anthropology and Gender Studies at Indiana University. Mary, an anthropologist and media scholar by training, focuses on how people’s everyday uses of technologies transform labor, identity, and human rights. Mary earned her PhD in Communication from the University of California at San Diego in 2004, under the direction of Susan Leigh Star. In 2020, Mary was named a MacArthur Fellow for her contributions to anthropology and the study of technology, digital economies, and society.
Mary’s work includes In Your Face: Stories from the Lives of Queer Youth (1999) and Out in the Country: Youth, Media, and Queer Visibility in Rural America (2009), which looked at how young people in rural Southeast Appalachia use media to negotiate identity, local belonging, and connections to broader, imagined queer communities. The book won the American Anthropological Association’s Ruth Benedict Prize and the American Sociological Association’s Sexualities Studies Book Award in 2009. And, with Colin Johnson and Brian Gilley, Mary co-edited Queering the Countryside: New Directions in Rural Queer Studies (2016), a 2016 Choice Academic Title.
In 2019, Mary co-authored (with computer scientist Siddharth Suri), Ghost Work: How to Stop Silicon Valley from Building a New Global Underclass. The book chronicles workers’ experiences of on-demand information service jobs—from content moderation and data-labeling to telehealth—work that is essential to the global growth of artificial intelligence and platform economies more broadly. It was named a Financial Times’ Critic’s Pick and awarded the McGannon Center for Communication Research Book Prize in 2019. The book was also awarded the 2020 Communication, Information Technologies, and Media Sociology section of the American Sociological Association (CITAMS) Book Award Honorable Mention. The book has been translated into Korean and Chinese.
Mary chairs the Microsoft Research Ethics Review Program—the only federally-registered institutional review board of its kind in Tech. She is recognized as a leading expert in the emerging field of AI and ethics, particularly research at the intersections of computer and social sciences. She sits on the editorial boards of Cultural Anthropology, Television and New Media, the International Journal of Communication, and Social Media + Society. Mary’s research has been covered by popular press venues, including The Guardian, El Pais, The New York Times, The Los Angeles Times, Nature, The Economist, Harvard Business Review, The Chronicle of Higher Education, and Forbes Magazine. She served on the Executive Board of the American Anthropological Association and was the Association’s Section Assembly Convenor from 2006-2010 as well as the co-chair of the Association’s 113th Annual Meeting. Mary currently sits on several boards, including the California Governor’s Council of Economic Advisors, Public Responsibility in Medicine and Research (PRIM&R), and Stanford University’s One-Hundred-Year Study on Artificial Intelligence (AI100) Standing Committee, commissioned to reflect on the future of AI and recommend directions for its policy implications.
At the end of the 18th century, Gaspard Monge introduced the optimal transport problem to understand the most efficient way of transporting a distribution of material from one place to another to build fortifications. In the last 30 years, this theory has found various applications in many areas of mathematics. However, more recently, optimal transport has also become a very powerful tool in many areas of machine learning. In this talk, we will give an overview of optimal transport, with some selected applications.
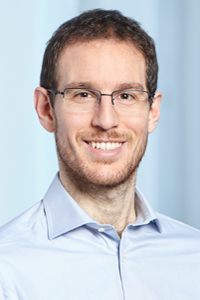
Alessio Figalli
Alessio Figalli earned his doctorate in 2007 under the supervision of Luigi Ambrosio at the Scuola Normale Superiore di Pisa and Cédric Villani at the École Normale Supérieure de Lyon. He was a faculty at the University of Texas-Austin, before moving to ETH Zürich in 2016 as a chaired professor. Since 2019 he is the director of the “FIM-Institute for Mathematical Research” at ETH Zürich. In 2018 he won the Fields Medal for “his contributions to the theory of optimal transport, and its application to partial differential equations, metric geometry, and probability”.
In October 2021, X officially became an option for gender on US passports. What are the computational changes necessary to adapt to this more inclusive gender option? In this talk, Meredith Broussard investigates why large-scale computer systems are stuck using 1950s ideas about gender, and what is needed to update sociotechnical systems. She explores how allies can leverage public interest technology in order to think beyond the gender binary, interrogate and audit software systems, and create code for social good.
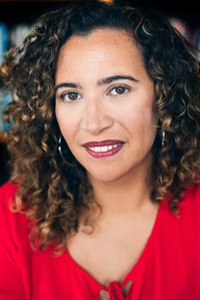
Meredith Broussard
In nature, groups of thousands of individuals cooperate to create complex structure purely through local interactions – from cells that form complex organisms, to social insects like termites that build meter-high mounds and army ants that self-assemble entire nests, to the complex and mesmerizing motion of fish schools and bird flocks. What makes these systems so fascinating to scientists and engineers alike, is that even though each individual has limited ability, as a collective they achieve tremendous complexity.
What would it take to create our own artificial collectives of the scale and complexity that nature achieves? My lab investigates this question by using inspiration from biological collectives to create robotic systems, e.g. the Kilobot thousand robot swarm inspired by cells, and the Termes robots inspired by mound-building termites. In this talk, I will discuss a recent project in my group – Eciton robotica - to create a self-assembling swarm of soft climbing robots inspired by the living architectures of army ants. Our work spans soft robotics, new theoretical models of self-organized self-assembly, and new field experiments in biology. Most critically, our work derives from the collective intelligence of engineers and scientists working together.
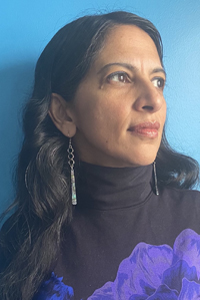
Radhika Nagpal
Radhika Nagpal is currently the Kavli Professor of Computer Science at Harvard University and a founding faculty member of the Wyss Institute for Biologically Inspired Engineering. Starting January 2022, she will be moving to Princeton University to lead new robotics initiatives. Nagpal leads the Self-organizing Systems Research Group (SSR) and her research interests span computer science, robotics, and biology. Nagpal was chosen by the journal Nature as one of the top ten influential scientists and engineers of the year (Nature 10 award, Dec 2014). Other awards include the Microsoft New Faculty Fellowship (2005), NSF Career Award (2007), Borg Early Career Award (2010), Radcliffe Fellowship (2012), the McDonald Mentoring Award (2015), AAAI and ACM Fellow (2020), and being an invited TED speaker in 2017. Nagpal is the co-founder of ROOT Robotics, an educational robotics company aimed at democratizing AI and robotics through early education; her lab's Kilobots have been commercialized with over 8000 robots sold worldwide. Nagpal is also the author of a Scientific American blog article on tenure-track life ("the Awesomest 7-year Postdoc", 2013), and is dedicated to creating a diverse and inclusive culture in STEM and academia.
Website: https://www.radhikanagpal.org