Invited Talks
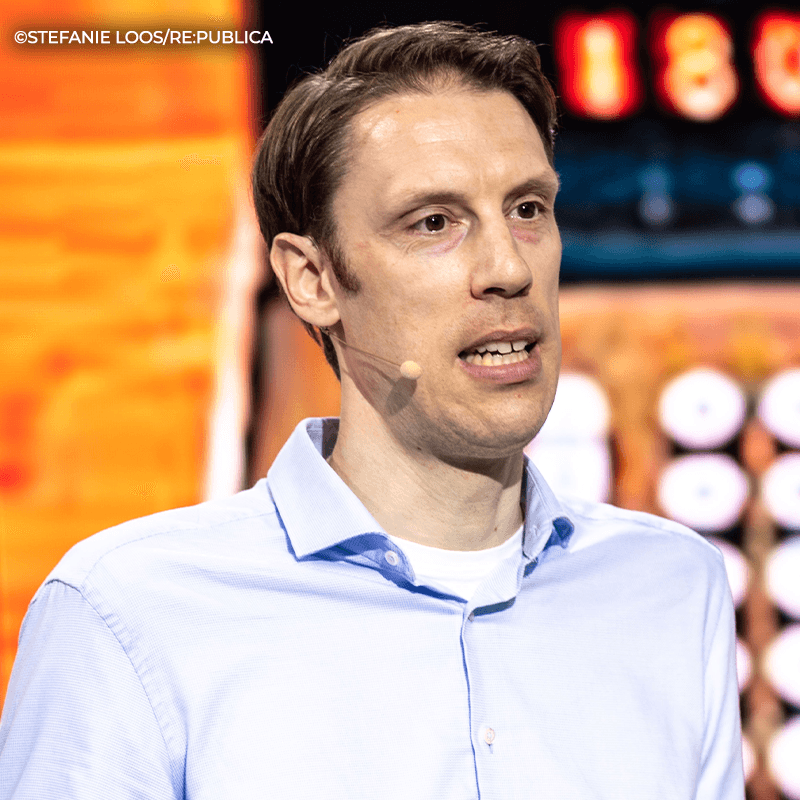
Björn Ommer
Björn Ommer is a full professor at University of Munich where he is heading the Computer Vision & Learning Group. Before he was a full professor in the department of mathematics and computer science at Heidelberg University and a co-director of its Interdisciplinary Center for Scientific Computing. He received his diploma in computer science from University of Bonn, his PhD from ETH Zurich, and he was a postdoc at UC Berkeley. Björn serves as an associate editor for IEEE T-PAMI. His research interests include semantic scene understanding and retrieval, generative AI and visual synthesis, self-supervised metric and representation learning, and explainable AI. Moreover, he is applying this basic research in interdisciplinary projects within neuroscience and the digital humanities. His group has published a series of generative approaches, including "VQGAN" and "Stable Diffusion", which are now democratizing the creation of visual content and have already opened up an abundance of new directions in research, industry, the media, and beyond.
Conventional machine learning paradigms often rely on binary distinctions between positive and negative examples, disregarding the nuanced subjectivity that permeates real-world tasks and content. This simplistic dichotomy has served us well so far, but because it obscures the inherent diversity in human perspectives and opinions, as well as the inherent ambiguity of content and tasks, it poses limitations on model performance aligned with real-world expectations. This becomes even more critical when we study the impact and potential multifaceted risks associated with the adoption of emerging generative AI capabilities across different cultures and geographies. To address this, we argue that to achieve robust and responsible AI systems we need to shift our focus away from a single point of truth and weave in a diversity of perspectives in the data used by AI systems to ensure the trust, safety and reliability of model outputs.
In this talk, I present a number of data-centric use cases that illustrate the inherent ambiguity of content and natural diversity of human perspectives that cause unavoidable disagreement that needs to be treated as signal and not noise. This leads to a call for action to establish culturally-aware and society-centered research on impacts of data quality and data diversity for the purposes of training and evaluating ML models and fostering responsible AI deployment in diverse sociocultural contexts.
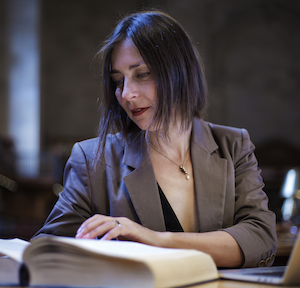
Lora Aroyo
I am a research scientist at Google Research NYC where I work on Data Excellence for AI. My team DEER (Data Excellence for Evaluating Responsibly) is part of the Responsible AI (RAI) organization. Our work is focused on developing metrics and methodologies to measure the quality of human-labeled or machine-generated data. The specific scope of this work is for gathering and evaluation of adversarial data for Safety evaluation of Generative AI systems. I received MSc in Computer Science from Sofia University, Bulgaria, and PhD from Twente University, The Netherlands. I am currently serving as a co-chair of the steering committee for the AAAI HCOMP conference series and I am a member of the DataPerf working group at MLCommons for benchmarking data-centric AI. Check out our data-centric challenge Adversarial Nibbler supported by Kaggle, Hugging Face and MLCommons. Prior to joining Google, I was a computer science professor heading the User-Centric Data Science research group at the VU University Amsterdam. Our team invented the CrowdTruth crowdsourcing method jointly with the Watson team at IBM. This method has been applied in various domains such as digital humanities, medical and online multimedia. I also guided the human-in-the-loop strategies as a Chief Scientist at a NY-based startup Tagasauris. Some of my prior community contributions include president of the User Modeling Society, program co-chair of The Web Conference 2023, member of the ACM SIGCHI conferences board. For a list of my publications, please see my profile on Google Scholar.
The world presents massive amounts of data for learning but the data relevant to any one thing or event is sparse. I will present evidence from the egocentric experiences of infants and young children in daily lives at home that demonstrate this sparsity, focusing on the case of early visual object recognition and object name learning. I will show how the statistics of infant self-generated experiences present solutions to the problem: learner control and optimization of the input, a developmentally constrained curriculum of spatial and temporal properties of the input, and the coherence statistics of individual episodes of experience. I will present evidence with respect to both low-level visual statistics and higher-level semantic categories. I conclude with a discussion of the alliance of the neural mechanisms that generate the statistics at any point in development and the neural mechanisms do the learning. I will the implications of the findings for artificial intelligence including studies using infant egocentric experiences as training data.
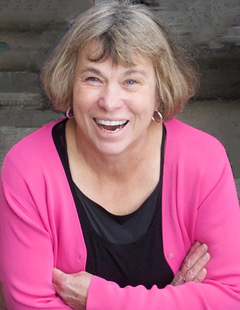
Linda Smith
Linda B. Smith, Distinguished Professor at Indiana University Bloomington, is an internationally recognized leader in cognitive science and cognitive development. Taking a complex systems perspective, she seeks to understand the interdependencies among perceptual, motor and cognitive developments during the first three years of post-natal life. Using wearable sensors, including head-mounted cameras, she studies how the young learner’s own behavior creates the statistical structure of the learning environments with a current focus on developmentally changing visual statistics at the scale of everyday life and their role in motor, perceptual, and language development. The work has led to novel insights about the statistics of self-generated experiences and their role in rapid learning and innovative generalization from sparse and limited experience and challenges current massive-data approaches in AI. The work also motivates her current efforts on defining and promoting a precision (or individualized) developmental science, one that determines the multiple causes and interacting factors that create children’s individual developmental pathways. Smith received her PhD from the University of Pennsylvania in 1977 and immediately joined the faculty at Indiana University. Her work has been continuously funded by the National Science Foundation and/or the National Institutes of Health since 1978. She won the David E. Rumelhart Prize for Theoretical Contributions to Cognitive Science, the American Psychological Association Award for Distinguished Scientific Contributions, the William James Fellow Award from the American Psychological Society, the Norman Anderson Lifetime Achievement Award, and the Koffka Medal. She is an elected member of both the National Academy of Sciences and the American Academy of Arts and Science.
'Sketches' of data are memory-compressed summarizations that still allow answering useful queries, and as a tool have found use in algorithm design, optimization, machine learning, and more. This talk will give an overview of some core sketching tools and how they work, including recent advances. We also discuss a couple newly active areas of research, such as augmenting sketching algorithms with learned oracles in a way that provides provably enhanced performance guarantees, and designing robust sketches that maintain correctness even in the face of adaptive adversaries.
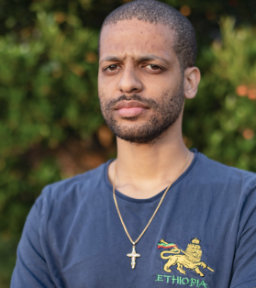
Jelani Nelson
Jelani Nelson is a Professor of Electrical Engineering and Computer Sciences at UC Berkeley, and also a Research Scientist at Google (part-time). He is interested in randomized algorithms, sketching and streaming algorithms, dimensionality reduction, and differential privacy. He is a recipient of the ACM Eugene L. Lawler Award for Humanitarian Contributions within Computer Science, a Presidential Early Career Award for Scientist and Engineers (PECASE), and a Sloan Research Fellowship. He is also Founder and President of AddisCoder, Inc., a nonprofit that provides algorithms training to high school students in Ethiopia and Jamaica.
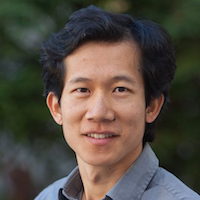
Percy Liang
Percy Liang is an Assistant Professor of Computer Science at Stanford University (B.S. from MIT, 2004; Ph.D. from UC Berkeley, 2011). His research spans machine learning and natural language processing, with the goal of developing trustworthy agents that can communicate effectively with people and improve over time through interaction. Specific topics include question answering, dialogue, program induction, interactive learning, and reliable machine learning. His awards include the IJCAI Computers and Thought Award (2016), an NSF CAREER Award (2016), a Sloan Research Fellowship (2015), and a Microsoft Research Faculty Fellowship (2014).
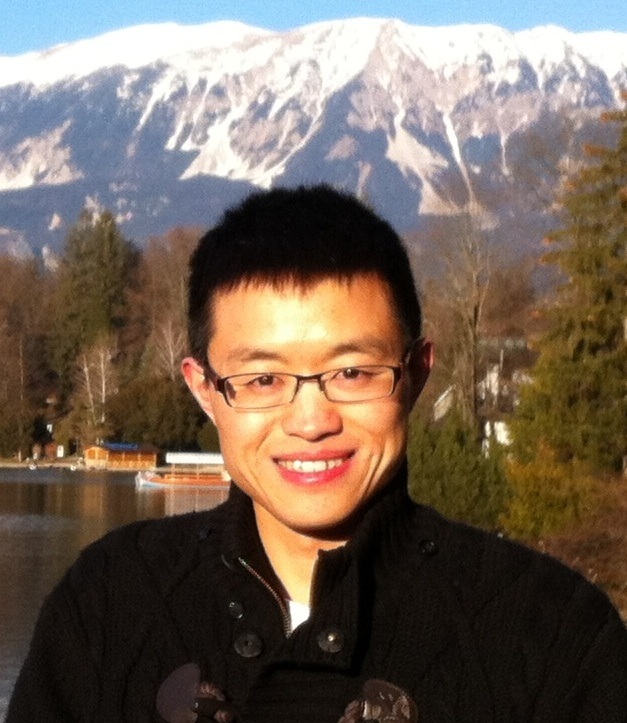
Jie Tang
Jie Tang is a WeBank Chair Professor of Computer Science at Tsinghua University. He is a Fellow of the ACM, a Fellow of AAAI, and a Fellow of IEEE. His interest is artificial general intelligence (AGI). His research received the SIGKDD Test-of-Time Award (10-year Best Paper). He also received the SIGKDD Service Award. Recently, he puts all efforts into Large Language Models (LLMs): GLM, ChatGLM, etc.
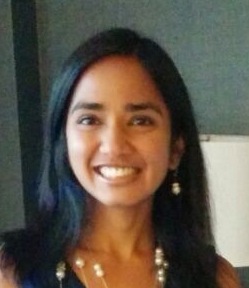
Aakanksha Chowdhery
Aakanksha led the effort on training large language models at Google Brain which led to the 540B PaLM model. Aakanksha has also been a core member of the Pathways project at Google. Prior to joining Google, Aakanksha led interdisciplinary teams at Microsoft Research and Princeton University across machine learning, distributed systems and networking. Aakanksha completed her PhD from Stanford University and was awarded the Paul Baran Marconi Young Scholar Award for outstanding scientific contributions in her doctoral thesis.
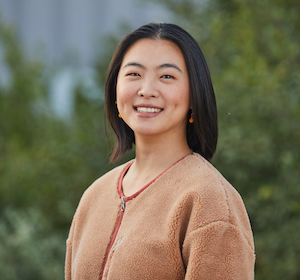
Angela Fan
Angela Fan is currently a research scientist at Meta AI focusing on large language models. Previously, Angela worked on machine translation for text and speech, including projects such as No Language Left Behind and Beyond English-Centric Multilingual Translation. Before that, Angela was a research engineer and did her PhD at INRIA Nancy, where she focused on text generation.
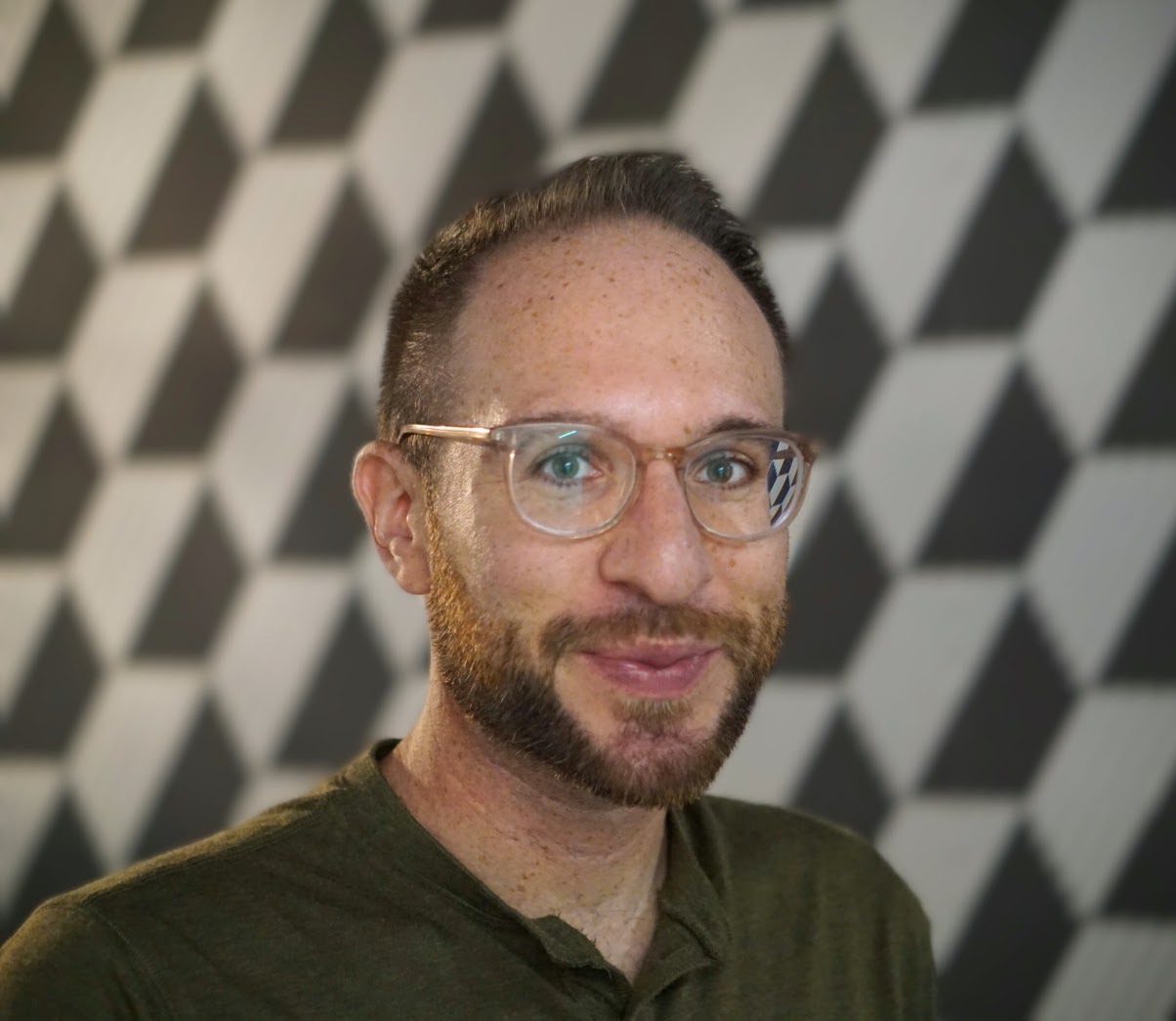
Alexander Rush
Alexander "Sasha" Rush is an Associate Professor at Cornell Tech and a researcher at Hugging Face. His research interest is in the study of language models with applications in controllable text generation, efficient inference, and applications in summarization and information extraction. In addition to research, he has written several popular open-source software projects supporting NLP research, programming for deep learning, and virtual academic conferences. His projects have received paper and demo awards at major NLP, visualization, and hardware conferences, an NSF Career Award and Sloan Fellowship. He tweets at @srush_nlp.
I'm a simple creature. I fell in love with foundation models (FMs) because they radically improved data systems that I had been trying to build for a decade–and they are just awesome! This talk starts with my perspective about how FMs change the systems we build, focusing on what I call "death by a thousand cuts" problems. Roughly, these are problems in which each individual task looks easy, but the sheer variety and breadth of tasks make them hard.
The bulk of the talk is about understanding how to efficiently build foundation models. We describe trends in hardware accelerators from a perhaps unexpected viewpoint: database systems research. Databases have worried about optimizing IO – reads and writes within the memory hierarchy – since the 80s. In fact, optimizing IO led to Flash Attention for Transformers.
But are there more efficient architectures for foundation models than the Transformer? Maybe! I'll describe a new class of architectures based on classical signal processing, exemplified by S4. These new architectures: are asymptotically more efficient than Transformers for long sequences, have achieved state-of-the-art quality on benchmarks like long range arena, and have been applied to images, text, DNA, audio, video. S4 will allow us to make mathematically precise connections to RNNs and CNNs. I’ll also describe new twists, such as, long filters, data-dependent convolutions, and gating, that power many of these amazing recent architectures including RWKV, S5, Mega, Hyena, and RetNet, and recent work to understand their fundamental limitations to hopefully make even more awesome foundation models!
A github containing material from is under construction at https://github.com/HazyResearch/aisys-building-blocks. Please feel free to add to it!
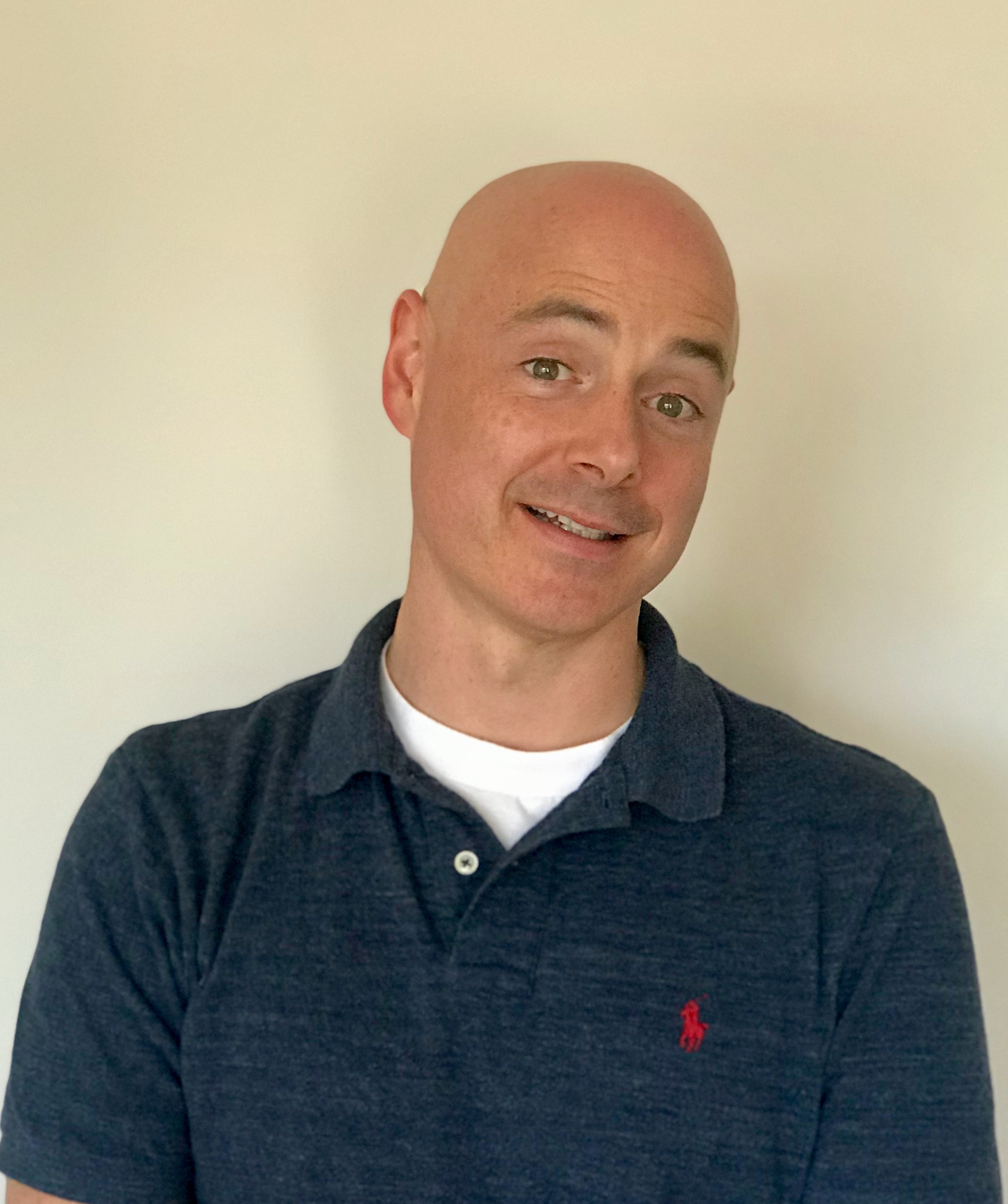
Christopher Ré
Christopher (Chris) Re is an associate professor in the Department of Computer Science at Stanford University. He is in the Stanford AI Lab and is affiliated with the Machine Learning Group and the Center for Research on Foundation Models. His recent work is to understand how software and hardware systems will change because of machine learning along with a continuing, petulant drive to work on math problems. Research from his group has been incorporated into scientific and humanitarian efforts, such as the fight against human trafficking, along with products from technology and companies including Apple, Google, YouTube, and more. He has also cofounded companies, including Snorkel, SambaNova, and Together, and a venture firm, called Factory.
His family still brags that he received the MacArthur Foundation Fellowship, but his closest friends are confident that it was a mistake. His research contributions have spanned database theory, database systems, and machine learning, and his work has won best paper at a premier venue in each area, respectively, at PODS 2012, SIGMOD 2014, and ICML 2016. Due to great collaborators, he received the NeurIPS 2020 test-of-time award and the PODS 2022 test-of-time award. Due to great students, he received best paper at MIDL 2022, best paper runner up at ICLR22 and ICML22, and best student-paper runner up at UAI22.
In this talk I will discuss first solutions to some of the challenges we face in developing online RL algorithms for use in digital health interventions targeting patients struggling with health problems such as substance misuse, hypertension and bone marrow transplantation. Digital health raises a number of challenges to the RL community including different sets of actions, each set intended to impact patients over a different time scale; the need to learn both within an implementation and between implementations of the RL algorithm; noisy environments and a lack of mechanistic models. In all of these settings the online line algorithm must be stable and autonomous. Despite these challenges, RL, with careful initialization, with careful management of bias/variance tradeoff and by close collaboration with health scientists can be successful. We can make an impact!
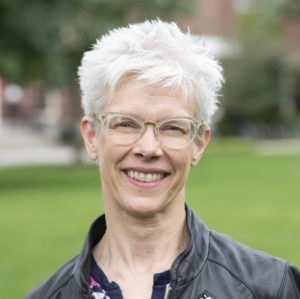
Susan Murphy
Susan A. Murphy is Professor of Statistics and Computer Science at Harvard University. Her research focuses on improving sequential decision making in health, in particular the development of online, real-time reinforcement learning algorithms for use in personalized digital health. She is a member of the US National Academy of Sciences and of the US National Academy of Medicine. In 2013 she was awarded a MacArthur Fellowship for her work on experimental designs to inform sequential decision making. She is a Fellow of the College on Problems in Drug Dependence, Past-President of Institute of Mathematical Statistics, and a former editor of the Annals of Statistics.